112: Give your salary data strategy a glow up
Welcome everybody. Thanks for joining us today. My name is Ruth Thomas. I'm Chief Evangelist and SVP of Marketing here at Payscale. So I help to drive our thought leadership and oversee our marketing teams through all the work that we do here at Payscale.
Speaker 1:Sarah, over to you.
Speaker 2:Good morning, good afternoon, good evening and good night. I'm Sarah Hillenmeyer. I've been building data and AI enabled tools in HR tech, medicine and other industries for more than fifteen years. At Payscale, I lead our AI and data insights teams within our research and development organisation.
Speaker 1:Thank you, Sarah. Okay, so for the last couple of years, I think we've run a short data thought leadership webinar series. So we're doing the same again this year. Really, we aim to bring you some key insights around how to manage data considerations for when you are creating a data strategy. We aim to bring together thought leaders, data experts like Sarah, practitioners, customers, partners to join us in that discussion.
Speaker 1:This is the first of those episodes of this year's series. We have another webinar coming up on May, where we will also be sort of building on the content that we've covered today. But in today's session, we really wanted to cover these things here. So whether you're pricing one job or whether you're pricing thousands of jobs, having consistency in terms of your approach to using data and how you use that data to support the business goals that you want to do is important. So we're going to start the session off sharing you with a model that we think about when we think about building a data strategy within an organization.
Speaker 1:And then there are some other hot topics that often come up in conversation as we think about building a data strategy. So data transparency, data biases and data and AI. So we're going to cover off those three in this session as well, sharing thoughts and kind of like where we're seeing in practice and some kind of key innovations that we're seeing starting to happen in the market. So that's the aim of today's session. So let's get started.
Speaker 1:I'm going to kick us off in terms of thinking about what makes a good data strategy. And we think about it as having three key components. So we're going to walk you through this Sarah and I. So the first is the input data, the data that you potentially purchase from providers or you get free from government sources or from the web. And Sarah is going to talk you through about the various things you should be thinking about as you bring, select that data.
Speaker 1:Then there's the kind of how are you going to use that data internally. So I'll run you through that, like what are the considerations you think about as you bring that together internally to support your compensation processes. And then in today's world of pay transparency, the discussion around what you should or should not communicate in terms of, you know, the data that you're using and how you're using it is becoming more pertinent. So we'll talk you through some guidelines and some kind of the things that we're seeing happening across our customer base. So I think Sarah, you're going to start us off here with questions to answer as you evaluate any data source.
Speaker 2:Great. When you're considering using a data source, whether it's a free data source or something that you're thinking about purchasing, these are the questions that I recommend answering and keeping track of. The most important question is whether the data source contains pay data about the jobs of your organization. You want to be able to find most, let's say 80% or more of the roles that you're trying to price within the data source that you're considering. We generally recommend that for each role you're pricing, you find a job in the data source that's at least a 70% match in terms of the skills, key responsibilities, experience, and reporting structure.
Speaker 2:The next thing to consider is the repeatability. How easy is it to price jobs in a consistent way year over year or time period over time period? Are the changes that you're seeing in the data that you're buying reflective of the market? Or is it a potentially noisy data source that's pulling from different populations at different times and showing you variation that isn't necessarily what you would want to bake into your merit increases and your market increases for your employees that year. Next up, this is more of a checkbox from my perspective, although we can talk about how to evaluate methodology and explainability.
Speaker 2:But any data source that you buy or use, you should understand where the numbers are coming from. How are they calculated? If they're calculated, if they're not calculated and they're collected empirically, where did the data come from? When was it collected? What happened to it from that point of collection to the point that it's in your hands?
Speaker 2:That's an important thing to know. Even if you don't feel like you can totally evaluate it, you should know the answer to that question. Number four is how old are the data? When were those data points collected? Have they been aged since the time of collection?
Speaker 2:Aging, if you haven't heard it, is a term that refers to adjusting the market numbers by some fixed percentage per month or per year to account for market trends that have changed the pay since the time that the data were collected. Number five is biases. We're going to talk a lot more about this later, but you should have a sense of the underlying biases in the data sample and how that might impact the way that you need to use that data going forward. And lastly, and Ruth will touch on this more, as you think through which data sources to use for particular tasks, think about who else can see the data. Is it something that your employees can access?
Speaker 2:That might change the way that you think about using that data and it might influence your compensation strategy and your communication strategy around that data versus having something that you know that your employees don't have access to. Ruth, can you talk us through the processes of how to deal with that data once you have it?
Speaker 1:Yeah, so you've got all that wonderful data, you've sourced your data. I guess the next step is what are you going to do with it and how are you going to use it internally? Probably the biggest decision point is how you're going to aggregate multiple sources if you're using more than one source of data. We're not going to talk today about the different types of data sources that are available. We will potentially cover that in another version or in another episode of this series.
Speaker 1:That's something you'd like us to cover as well, leave some comments in the Q and A and we can make sure that we cover that in more detail. But there are lots of kind of considerations in terms of aggregation, in terms of potentially, what percentile are you going to use within each of the survey sources that you're using? Are you going to weight them differently? So do these sources have more importance or less importance for you? And does that change by the different talent groups that you have within the organization?
Speaker 1:How are you going to deploy? How are you weight? Which is more important for you? Sarah mentioned ageing, so you know as you bring the different data sources together you're going to need to think about your aging strategy so that you're looking at them all essentially from the same time frame as much as is possible. And then you know are you going to use the same methodology for aggregation across different job groups or different talent groups?
Speaker 1:So that's an important question because you may have talent groups that you know, where there is a high demand for talent or that the labor market is moving very fast or there is a hot demand for certain skills. And so therefore, how are you deciding to use data to think about pricing jobs, to think about building pay ranges, maybe slightly different for that group than for another group where they may be sitting in a more potentially stable talent market. And the same when it comes to thinking about geo differences as well as like how are you going to factor that into bringing all these data sources together. So that's probably the most important step here in terms of developing your internal processes. But you also need to think about how you're going to handle the data that you're bringing in relative to what exists in your organization today.
Speaker 1:So you may have some old ranges around within the organization. They may be job based or grade based. What are you going to do with this data relative to those? And then also you really need to do that comparison, which I know you will do. It's that comparison of the market data with your current incumbents.
Speaker 1:But you may need to sense check that a little bit as you refine the data sources that you're choosing and the data sources that you're aggregating and bringing together. Obviously many people will use the market data to help build pay ranges. Again, you need to think about what your strategy is for that and is that going to be consistent across different talent groups. And then today's age, obviously many of us are using the data that we get from these surveys to post in job postings in order to comply with pay transparency legislation. So how are you going to go about calculating the range that you'll actually list in the job posting?
Speaker 1:Again, not something we're going to cover in-depth today. We have a detailed webinar that talks about that whole process of selecting job postings. I'll ask Amber to include that link in the follow-up. But it's really like I have my market data. I've made it, I've made some discerning decisions about the data I'm bringing into the organization.
Speaker 1:And then thinking about that theme of consistency, how am I going to apply that in terms of how we will use it within the organization? I don't know if there's anything you wanted to add there, Sarah. No. No. Okay, cool.
Speaker 1:And then the third step in our model, we said, you know, in the age of pay transparency is thinking about what you're actually going to communicate. So previously, I mean, I've worked in compensation for many years. This was something that we did in a back room somewhere. Nobody got to know what we were doing. And probably the only interaction point, for example, a line manager might have with the market data was potentially through the merit review process or a discussion during the hiring process.
Speaker 1:There is a demand, you know, to make conversations more two way and to make conversations more meaningful about pay for people to be able to understand where you are potentially sourcing market data. So I'm sure many of you on the call have been through the experience of having an employee or potentially a candidate through the hiring process come to you and say, well, this is the data that I'm seeing for the job. Know, in order to be able to counter that, you need to be able to have a conversation about the different types of market data sources, potentially where they're sourcing that data from. So that might be job boards or Google even nowadays, you know, that's very easy through Google to get that data for a job relative to how you're choosing market data. And that's going to force you in a way then to be more transparent.
Speaker 1:And so that's why organizations are starting to think more about, you know, how far do we go in terms of sharing data? So, you know, what is it you're going to share? You have various options. Are you going to share the methodology? Are you actually going to share the market data ranges?
Speaker 1:That's probably one people feel, you know, least likely to share. And then are you going to tell employees potentially where they sit against that range? And then are you going to explain the data sources that you've used and why? That's probably quite technical conversation for most of your audience in your organization. But there is probably a way that you can tell that story in terms of, you know, a more generic level.
Speaker 1:It's like we choose market data sources that represent X, Y, Z and we use that, you know, to do this. So having a talk track to, you know, the type of data sources that you're using without going through a highly technical soliloquy about how you, you know, selected all of those processes per like the stuff that Sarah talked to through at the beginning. And then, just thinking about what more do you need to do? So what regulations apply to us as we think about communicating pay and what are our competitors doing in terms of how they communicate about market data as well. So an interesting conversation, interested to hear your thoughts, interested to hear your questions as we move into the Q and A about how comfortable you feel at this point about communicating market data and what elements of that you think that you should be communicating.
Speaker 2:And we'll talk more about different places to land on that transparency spectrum and how to migrate from one section to another if you want to increase your transparency. But just to wrap us up here, when we say data strategy or compensation data strategy, those three pillars are the things that we're talking about. Which input data are you using? Does it cover your jobs? Is it a repeatable data set that has stability in it where the trends are real?
Speaker 2:Can you explain it? Is it fresh? Is the bias something that you can work with? And again, we'll talk more about that. And then how do you process that data to generate pay decisions for all of your employees?
Speaker 2:And then thirdly, how do you communicate that? And obviously, these three pillars are interdependent, but we think it's useful to think about each of them as separable. You can think about each of these separately, even though the data choices you make may influence your communication strategy or vice versa. If you're starting out, just start marching down this line. Pick your data, think about your process, determine your communications.
Speaker 1:Yeah, so this is a great reference slide for you. You obviously will get a copy of the slides in the follow-up communications that will go out, but this is probably, you know, is where we pull together that model for you. A good checklist slide for you to take back to your own organisations. Okay, so we've brought up the topic of data transparency. Let's dig into that a little more.
Speaker 1:I talk a lot about pay transparency. You would have to have been living somewhere very strange to not know if you're working in the field of compensation and HR that transparency is a thing. It's driving a lot of what we do and decide around compensation practice today. And that's obviously particularly been driven by legislation. And that legislation is really around promoting fairness, equity and trust by ensuring that employees understand how their pay is determined and how it compares to pay of their peers.
Speaker 1:So that's obviously about openly sharing information and we know many of you have been on that journey. We see that in our results from our annual compensation best practice study. And also through the webinars that we do, where we poll you, we're gonna go up on our polls coming up in a minute. But we know, this is a key activity that you've been working on and thinking about how you communicate pay is top of mind. But we often talk about that in the context of communicating pay.
Speaker 1:So I'm going to share with you the pay transparency continuum that we often share here at Payscale. And then Sarah is going to say, you can take that and you can do it for data too. So, when we think about the pay transparency continuum, there's two ends to this where you're very secret about pay. And probably when I started my career in compensation, that was very much where we were. And then at the far end of the spectrum, you've got like being very transparent, fully transparent, potentially publishing all your salaries publicly.
Speaker 1:There are very few organizations that do that. Where we see most people falling is in the share salary range data for the current role with the employee. So that tends to be the goal where most organizations are trying to get to. There's obviously quite a lot of work that needs to happen to get you to that point. But as we think more and more about career progression, then the point in the range where we have all salary range data shared internally, so people have an understanding of how their career can progress, how their pay can progress, then having that wider view of just not their own pay range, but maybe the pay range of the next roll up or the next roll up will give them an idea around career and pay progression that's important as well.
Speaker 1:So we've talked about this many times here at PayScale. This is our pay transparency continuum, but we're going to slightly tweak it and you're going to talk us through how that might apply to a data strategy, Sarah.
Speaker 2:Took the continuum. I love this spectrum analysis. And I put the data strategy on top of this. So instead of thinking about sharing pay on this continuum, we're thinking about sharing or talking about sharing how the ranges are created and how those decisions are made and that decision about how someone's aid is reached. At the pay secrecy end of the spectrum, you have no information at all.
Speaker 2:You know your pay, but nothing about how it's created. I would say at this end of the spectrum, you want at least your comp team and probably your executive board to understand that compensation strategy. That might mean having it written in some place, or having a set of guidelines that everyone who's working on compensation is following so that you know that everything's happening the same way across different practitioners, but they're not necessarily sharing that with managers and certainly not with employees. On the other end of that spectrum, have the full information. If you had written down your comp strategy, here's what it would say.
Speaker 2:It's which surveys you're using, what jobs you match to, what other jobs are in a comparable group, how the range was created from that market data. And along the way, we have a couple of other stop points. You can share sort of high level philosophy with your managers and potentially your employees, or you could share the strategy itself with managers and employees, even if you don't share each data point. So there's lots of different places to be here. Right now, we're seeing folks moving from the data strategy secrecy into more of the philosophy sharing, especially with managers and sometimes with employees to help drive those better conversations that Ruth was talking about.
Speaker 2:Where it came from helps understand how I can impact that and what sort of levers I have to pull to get paid more or to grow in my career.
Speaker 1:So a good takeaway for all of you on the call today is to think about where am I on those two continuums of pay transparency? And where might I aspire to be? What do I have to do to get there? And a lot of that will be influenced by kind of your overall culture of transparency within your organizations. But as Sarah alluded to there, we know there's a growing expectation for stronger conversations around pay and how pay is determined.
Speaker 1:And we need to really kind of equip ourselves and equip managers who are frontline facing those questions and those conversations around, you know, how the pay that an individual has been derived. Okay, so we're going go move to our first poll. Let's find out how transparent you are with your data strategy. So in the poll tab, you're going to be able to vote there on a number of options. So do you communicate your data strategy in terms of how you're using market data only with your HR or senior management to managers?
Speaker 1:Do you communicate to everybody? Are you in the move towards transparency bucket or maybe you're not transparent yet? Or you maybe you potentially don't know. So we're going to let you vote away there. It's really interesting for us to see how you're thinking and how this is evolving.
Speaker 1:It's important for us, Sarah, I guess, as we think about supporting organisations in giving them the data sources that we do and the choices that they're making there.
Speaker 2:Absolutely. We work with customers that are in many different places on the spectrum and understanding what the pain points are and what they need from the software and the data to to help push in the direction that that's aligned with their goals is really helpful. So these kinds of polls and talking to customers and prospects to understand these pain points are very valuable information for us.
Speaker 1:Okay, so I could see people voting away, but I think we're leveling off. Yep, Amber's sharing the results. Thank you, Amber in the background. Right, so this is probably in line with where we were talking about where people were on those continuums. So we have the majority of you are really communication to HR or senior management only, but there is a good body of you, 19 and a half percent of you nearly who want to move towards transparency.
Speaker 1:And we see a number of you who are communicating to managers only, because we said, know, they're really important participants in this process, but a good number of you, 14% communicating to all within the organization. So some of you are on your journey. Some of you are probably in that middle part of the journey that we, that we shared earlier. Okay, now another question we often get asked is around data bias, because there's this common myth Sarah that there is one market number out there and there is one data source that you can go to and it's going to give you that magic number and that is going to be the right number of what someone would be paid. And I love the way you explain data bias and why that is not the case.
Speaker 1:So I'm passing it over to you.
Speaker 2:All right. The unicorn myth of having the exact answer is just there if you could only find it. I don't mean to be inflammatory with this, but all market data is biased. Everything that you're considering, buying, purchasing, looking up on the internet has some form of bias. And I'll talk you through some of the more common ones and talk you through how to handle that bias and when to think about it as a big deal and when to just sort of know that it's there.
Speaker 2:Starting at the top end of what you might pay for, surveys, compensation management surveys, and data sources like Peer are generally collected from big companies. This is because bigger companies are likely to participate in these surveys and pay for these expensive data assets. And I'll show you exactly what that SKU looks like in the next slide and talk to you about what that means. Other data sources that you might be using, like PayScale's employee reported data or information from Glassdoor or Levels. Fyi, tends to skew towards younger, more technical respondents.
Speaker 2:There you see more 25 year old software engineers taking out those surveys, filling out that information than you do 70 year old accountants and more than you do blue collar worker working in a union job. So the data that comes out of that is aggregated and massaged, but it represents that younger, more technical population more heavily than it does some of the other populations that you might be hiring for. Interestingly, as more and more states have added job postings, job pay transparency requirements and legislation, and more and more job postings have pay information, I've been watching the aggregate job posting data shift a little bit here and there. As states that generally have a lower cost of labor add pay transparency legislation, I see the market range at the national level drop for a job. And then as a state where the cost of labor is very high, as transparency legislation, I see the market range for the national level go up a little bit because the underlying data mix has shifted as those different states change their laws and as different companies are then adding data into that aggregate data pool.
Speaker 2:So big plug for the Bureau of Labor Statistics here. They do a really, really great, very statistically sound job of evening out both labor pool distribution data as well as pay data. So if you're not checking the Bureau of Labor Statistics website every once in a while to see what their take is, I absolutely recommend it because it'll give you a much cleaner, unbiased view of labor in this country, at least, and of pay across different jobs in different professions, at different industries and locations. The downside is it's pretty tough to build a compensation strategy and plan based only on the Bureau of Labor Statistics data because it's not granular enough and it's too old to be super relevant. But it's a really good, free, easily accessible reference point.
Speaker 2:So just to pull the covers back on this a little bit, I'll show you some of the biases in PEER. And I'm using PEER here, one of PayScale's data sets, as a representative of a participation based aggregated data set. So this is my guess is this is a lot like what the data behind a third party survey would look like. I happen to have access to this one, so I want to show you here, but apply this thinking to any of the large survey providers that you're considering publishing. On the x axis here, what I have are different size bins.
Speaker 2:So each of these bins represents companies of particular size. On the left hand are companies that have less than five employees, and on the right side of this axis is companies that have at least a thousand employees. What the y axis is showing is how much of the workforce is represented by people working at that size company. So Bureau of Labor Statistics is medium blue. That's the one I trust.
Speaker 2:What you can see here is that about 8% of The US workforce works at a really small company, less than five employees. They could be self employed. They could work with two people at a very small firm. And about 18% of The US workforce works at a company that has a thousand or more employees. So there's kind of this dip in the middle that shows the trend over various company sizes.
Speaker 2:But you see quite a few people work at very small companies and less than 20% work at these really large firms. When we look at peer, and again, I'm using peer as a representative here, we see that more than 90% of the incumbents that are included in the peer data set work at big companies. So we are overrepresenting data from very large companies here compared to The US labor force in general. The upside is if you work at a company that has a thousand or more employees, the data that's in PEER or a third party survey that's collected in similar way is very representative of what pay is at those larger firms. The downside is if you work at a small firm, if you work at a 20 person company, using those data assets and data sources may not give you a very clean reflection of what pay would look like at that smaller company.
Speaker 2:And you may need to go look at Bureau of Labor Statistics or other places to understand how that large firm pay relates to what it would look like at a smaller organization that you're representing.
Speaker 1:Oh, sorry, sorry. I
Speaker 2:wanted to point this out and I won't go into as much depth, but like other surveys, peer is also biased towards particular industries. And this can be very meaningful to folks, and sometimes it's not. But if you're using an all, all, all data cut from peer or from a different survey provider, it's important to understand what types of incumbents and organizations are making up that all, all, all data cut. For a job like a compensation analyst, and we probably have some on the phone, you may already know this, but compensation analysts make a little bit less in colleges and universities and slightly more in health care, But the ranges are quite overlapping and really industry doesn't impact the pay of a compensation analyst very much. We see compensation analysts get hired from one industry into a position in another industry and then move back to a third industry all the time.
Speaker 2:The talent pool has a skill set that's useful to lots of different companies. On the other hand, a job like a project manager makes significantly more money in construction or in an architecture firm than they do in finance. It's almost like it's a different job, but it's called the same thing. Like 40% more if you work in architecture than in finance as a project manager. So if you are pricing jobs and you pick up a survey that is mostly architecture and construction, but you're trying to price jobs in finance, you could end up with a view of a project manager role that says it should be paid significantly more than what the market rate actually is in finance, even though it kind of looks okay for Comp Analysts.
Speaker 2:So something to be aware of and something that we can tell you how to handle. But basically every data source you get will have a bias like this, where some industries are more represented and some are less represented. So what you're
Speaker 1:doing is to make sure that you discuss with your vendors on that. So you're going to talk us through this, I think.
Speaker 2:Absolutely. Just ask ask your vendors. I'll I'll send you that file if you wanna see it for peer, and understand where those those data are coming from. And my guidance here is if you're using an all all cut, which I love that there's lots of data in there, that's a robust thing to do. If the bias of the underlying dataset doesn't match the firmographic details of your organization, you could have a problem.
Speaker 2:The way to combat that problem is to be more specific, to use a specific scope or data cut that is oriented towards firms like you. So even if you're using a data set like Peer and you know it's got a lot of healthcare and retail in it, if you're in materials and mining or you're in finance, trimming down that list and looking specifically at organizations that are like you will help make sure that the bias of the underlying data set isn't impacting your market ranges and sending you often into a range that's really not appropriate to the labor pool that you're hiring from. Occasionally, there's not data available for the industry that you're really interested in. And so what we see folks do there is use some kind of adjustment factor to say, well, I'm trying to price a dentist in the construction industry. There's no other dentist in the construction industry, but I know that dentists in the healthcare industry make a little bit more than they do in other industries.
Speaker 2:There are actually dentists in other industries, even though it doesn't sound like there would be. They're dentists and insurance. And I know that they make a little bit less. So I might take that overall number and say, I think it's probably less than health care because my industry is likely to pull more from talent that would also work in the insurance industry or similar than it is to pull dentists that work in the health care industry. So you can make some adjustments.
Speaker 2:And comp pros that have been doing this for a long time often have a back of the envelope way of doing this for lots of different scenarios.
Speaker 1:Okay, so we've covered data biases. We'd like to hear if this is something that you've been thinking about in terms of your data strategy. So we have another poll going in the polls tab. Do you monitor your data processes for bias? Is this something you do continuously, occasionally, you're planning to, you weren't aware of the issue and hopefully you are now after Sarah's great explanation or you don't know.
Speaker 1:So we're just interested to see if this is something that resonates with you and something that you're thinking about as you work with different data sources. And I can see you're busy voting away there and I can see some questions coming in. So we are going to move to the live Q and A soon. So any questions you've got specifically about the biases that we've talked about so far about transparency, get those into the Q and A and Sarah and I will be able to address those very shortly. We have one more topic to cover before we move into the live Q and A.
Speaker 1:But let's have a look at what you are saying about do you monitor your data processes for bias?' So occasionally, so we've got nearly 38.5% of people saying they occasionally monitor and we've got 20% of you who are saying, nearly 20% of you saying continuously and then some of you who are planning to do it. So it's good to see, I think we've got a group of mature compensation practitioners here on the line. This is something that's a topic that you're aware of. Hopefully this is just a good refresher on why it's important to think about those biases as you work with your data sources. So thank you very much for responding to that poll.
Speaker 1:Okay, so you can't talk about anything to do with HR or anything to do with anything today without talking about AI, to be honest. And we've been talking about AI as it relates to data and data strategy, as we've done this webinar series over the last this is the third year we've kind of run it. And there's definitely been a huge move in terms of progress, I think, Sarah. We've been thinking about it over that time very actively. We've been thinking about it in terms of how we incorporate it into the data solutions that we have here at Payscale.
Speaker 1:But Sarah is going kind of talk you through where we're at today in terms of how you should think about AI as part of your data strategy.
Speaker 2:Yeah, as Ruth said, although I've been doing AI since before it was cool and PayScale's been doing AI since before it was cool, 2023 was the year that generative AI exploded into the public discourse. And in 2024, we saw the use of AI in HR kind of pop up and start to go more mainstream. This year, HR professionals are embracing AI, particularly for tasks like compensation management and job description creation. As part of an annual poll that we do called Compensation Best Practices Report, which you can get to via this link, we gather responses from 3,600 comp and HR professionals a mixture of PayScale customers and also folks that don't use our tools. This year, we found that 71% of those respondents have a positive sentiment about using AI for market pricing.
Speaker 2:And similarly, a growing majority of respondents are interested in AI for other use cases, like recommending pay increases, helping support legislative compliance, and for documentation and communications. In the compensation world, as I alluded to a little bit earlier with my back of the envelope calculations, data driven techniques to extrapolate from existing data are our bread and butter. Many of the methods that are common in our field, taking a weighted combinations of underlying data sources or aging the data or calculating and using differentials, are really just simple implementations of AI. Even doing a Google search is basically using AI. So in the now and certainly in the future, I expect that AI tools, enabled tools will make these methods that we have been using for years more robust, more scalable, more repeatable, and more accessible to folks that don't have years and years of expertise and know the data inside and out.
Speaker 2:With tools like Explore, which we launched this week, and the underlying AI powered data asset, we're using AI to find the same patterns that we've all been measuring and using as part of the art of compensation. But leveraging AI and the data at scale to do it instead of doing it one off each with their own methodology. This helps us correct the biases in the underlying data sources, and it improves the accuracy of calculating a pay market range when the data are sparse. So if you want to hear more about this, connect with me on LinkedIn or schedule a demo and we'll show you what those tools look like. The same techniques also help us discover some patterns that are pretty interesting and insights about pay and compensation strategy that haven't been measurable before.
Speaker 2:So compensation decision makers, whether that's a compensation analyst, an HR generalist, a VP, an exec, or a board need education on how artificial intelligence can make compensation related decisions more accurate and more efficient. AI can assist employers with job management, including writing and analyzing job descriptions. And AI is also being currently used and more tools coming for market pricing and pay analysis. Over the next couple of years, all of these boxes that don't have stars, I expect to see more and more areas where AI makes our existing workflows more efficient and helps us uncover key insights that can drive our compensation strategies. I will say the potential for AI to extend bias rather than mitigate it is still a very real concern, especially where AI can unknowingly perpetuate historical salary discrimination and pay disparities for protected classes.
Speaker 2:Ultimately, human intervention is required to ensure that AI powered algorithms are properly informed and producing fair outputs. I like to say that AI can scale bias or reduce bias at scale, But our judgment and our expertise helps make sure that we push towards that reduced bias at scale rather than the scale bias. I also want to flag for folks that are doing this work day in and day out, pay gaps are not excused by the reliance on flawed models. If an employee in this country, if an employee can demonstrate that they are paid differently from another employee for equal or similar work, the burden shifts to the employer to demonstrate that that pay differential is due to a permissible reason, like differences in skills or differences in performance or differences in location or responsibility, rather than conscious or unconscious bias or discrimination, even if an AI tool made that recommendation. So employers, not the tool, are legally responsible at this time.
Speaker 1:Great, yeah, thanks for calling that out, Sarah. Something I was going to pick up on. So I mean, for me, as I think about it as a compensation practitioner, there are more data sources available today and the level of compensation decisions that we're needing to make are more granular. When I think about the whole skills conversation and how that plays into this, really, you know, having advanced decision support was the only way we were going to get to the place where we could start to correlate all those different information sources and information data points. We do need to get comfortable as practitioners in terms of thinking about how AI can support us in that process and can help to improve the decision making that we are doing whilst being aware all the time about what type of AI is being used.
Speaker 1:So if you're talking to a vendor and they're talking about AI, ask them how it's being deployed, make sure you understand how it's being used and in what way so that you can make an informed decision and feel comfortable about that as you move forward. Okay, so last poll before our Q and A poll. How are you using AI today? We know there's quite a few different use cases that people have. We've surveyed on this before in our compensation best practice report, but interested to see how prevalent the use of AI is in terms of the work that you're doing today.
Speaker 1:So I'm not going to read out all the options there. If you want to vote away, I think you can select all that apply in this particular poll. But are you using it in benchmarking, monitoring for pay equity, collecting intelligence on skills for recruiting, writing offer letters, using it to speed up survey participation, that would be nice and year over year updates. How are you using it today? And then we will move in to the much promised Q and A.
Speaker 1:I can see a few questions in there. So we'll be getting to those very shortly. Okay. Are we levelling out on votes? Okay, none of the above or unsure seems to be the majority at the moment.
Speaker 1:Of course, that may be the unsure category. So there has historically been a lot of AI. It was just probably just called machine learning at that point in market data sources. So I'm pretty sure that if you're using any form of market data sources, it's got some form of AI in there. And it just, you know, you may not just know it under the term AI.
Speaker 1:We can see people are also using AI to collect intelligence on skills for recruiting, upskilling, career pathing and or compensation. So there was nearly quarter of you using it for that. And then some of you using AI to benchmark, about just over 10%, nearly 12% of you using AI to benchmark and price jobs or predict pay ranges. So clearly an area where this is, I think we're going to see the growing influence of AI, Sarah. Don't know how do you feel when you look at those poll results?
Speaker 2:It's interesting, you know, and I think if I pulled my team, which is all people who build AI tools, we would get a similar distribution of places where people are seeing the value already and places where it's still kind of hype and like, I don't know. And then as you mentioned, Ruth, places where the old fashioned ways are actually not very dissimilar from what we think of as AI. We talk about pay equity, for instance, that's been based on regressions for many years. Regressions fall into the machine learning and AI umbrella because it's predicting things given data. So this is really interesting to me.
Speaker 2:And certainly the collecting intelligence on skills, career pathing and compensation is an area where AI, especially the next generation text processing from large language models can really make a big difference in the kind of information that is available to us. So fascinating to me to see the spread and the different places where people are interested or already using AI tools.
Speaker 1:So yeah, thank you for sharing all your insights, everybody, through those three polls. So closing us out, really, you know, the key messages that we wanted to get across today is, you you need a data strategy. And the aim of that data strategy is really to go for consistency of methodology so that you have a repeatable process that makes sense for your business. Aiming for transparency becoming increasingly important, you know, you're going to have more demands on you to be able to explain how you determining each employee's compensation and how that number was derived either from managers who are having conversations with employees or directly with employees themselves. So you think you getting comfortable with how transparent you want to be about the type of data sources that you're using as a journey I think you need to go through.
Speaker 1:Do you want to cover off the last two, Sarah?
Speaker 2:My goal today was to flag the biases issue and to show you that it's not necessarily a bad word, that all of the data assets that you consider are biased in some way, and that there are things that you can do to mitigate that bias and to work with it rather than against it. And then to highlight a couple of key places where we're seeing AI deliver value to practitioners like you and have you listen to each other a little bit through that poll to get an idea of where those tools are moving. If you're interested in hearing more about AI tools, drop a note in the comments. We could do another webinar that's more focused on that, either from a market landscape or thought leadership perspective.
Speaker 1:Okay, all right. Thank you. Right, we're going to move into Q and A now. There is another poll running in the background. If you would like to find out more about how Payscale can help you address some of the issues that Sarah and I have talked today, learn more about the AI innovation journey that we're on here at Payscale, then if you click in that poll, someone will reach out to you to get back to you to help you understand that.
Speaker 1:We're going to jump into the Q and A. I'm going to go back to one of the first questions that was asked of us, which was when we were really talking about transparency. And it says: Do you recommend employers having a written codified comp data strategy versus just something you used in practice? Classic consulting answer would be it depends and I think it really does depend. I'd say that depends on the size of your organization.
Speaker 1:If you have many compensation people and HR people kind of working on this together across the organisation, then quite often having some kind of written data strategy that people can refer to would be useful. If you're just like a sole person on your own and you're doing this as the comp analyst on your own and you're working with your HR team, probably useful to train them on it. But do you necessarily need something in writing? Probably not, I would say. So it really kind of depends on how many people you're trying to ensure that consistency applies to.
Speaker 1:It's like what is right for you to ensure you're applying the consistency and the decisions that you've made around how you're going to approach data. Now Sarah, there are a couple of questions about adjustments in there.
Speaker 2:Yeah.
Speaker 1:Do you want to take those two and talk to those two?
Speaker 2:I will. So we got two questions that are related. One was, do you have any recommendations for selecting the appropriate adjustment percentage for difference in industry? And the other was, what advice would you give for making adjustments where there isn't applicable industry for those who are newer to comp? I'm gonna give you two answers.
Speaker 2:One is go get a demo of Explore because we take the hard part out of it for you. And we've got lots of experts that do this at a job by job level so that you don't have to be an expert to understand how to do it. But I'll tell you how to do it yourself, too. The biggest piece of advice I can give you here is don't think about the industry that you're working in for a particular role. Think about the industry or industries that you're hiring from.
Speaker 2:So if you're hiring, accountants are a good example, cop analysts are a good example. If you look at your books and you say, Okay, my employees that are in this role came from a large number of different industries. And when I post this role, the next few people I'm hiring could also come from education or construction or retail or technology. Then in that case, an all, all, all data cut, no industry differential is going to give you the best representation of what pay is for that role, because it's not very dependent on which industry you're in. On the other hand, if you are pricing jobs or a family of jobs within your organisation where you are consistently pulling from one industry that is where you're always hiring from that industry and you would never consider hiring from a job outside of that industry.
Speaker 2:Then there's kind of two branches in this decision tree. One is to think about whether the job itself is particular to the industry that you're hiring in. So if you're working in agriculture and you're hiring a agricultural laborer, all of the data from any survey that shows you what the pay is for agricultural laborers is basically going to come from agriculture. It's not going to come from other industries.
Speaker 1:I've lost your sound, Sarah, I think. Yes. I'll just check whether it was me or you.
Speaker 2:Can you hear me now?
Speaker 1:Yes, you're back.
Speaker 2:Okay, great. I have a little bloopity bloop. So in that case, the job itself is representing the pay in the industry because it's specific to the industry. The trickiest part is when you have a job that's represented across many industries and the pay varies across those industries, like a project manager that I showed before, where they make 40% more in construction than they do in finance. In that case, what people generally do, and this is why I'm so excited about Explore, but what people generally do is they look at average differentials across lots of jobs and say, well, generally jobs pay a little bit more in this industry than they do in this industry.
Speaker 2:So if I'm pricing in finance, I might pay 8% more than in the construction industry because most jobs pay a little bit more in finance. That doesn't work perfectly, as this example shows, because some jobs pay more in one industry and less in another industry. But that's generally what the best practice has been until the tools have gotten better and caught up with being able to learn more job specific information about how industry affects pay. So let me try to sum it up. I know that was a lot of information.
Speaker 2:One is in many, many cases, you don't need to worry about industry at all because you're pulling talent from across industries and you're basically paying a market rate that is not dependent on an industry. That's case one. That's probably 70 to 80% of the jobs that you're pricing. Case two is you're pricing a job that's only found in a couple of industries and all of the market data that you're looking at is coming from those industries. They don't need a differential.
Speaker 2:That's probably 10%. So now we're at like 80 to 90% of the data, no differential. That last 10 is the hard one where the pay varies across industries and the data that you have isn't totally represented by the industry that you're pulling from. And then that's where the art part comes in. And I think AI can make a really, really big difference.
Speaker 2:But the general practice over the last several years has been to look at what I call a job agnostic differential of how does pay generally differ between these two industries. You can get that from a data asset like PayScale has, or you can look it up on BLS and you can see how pay varies across industries.
Speaker 1:Okay, thank you for that answer to those two questions, Sarah. I think maybe we've got time for one more question. Someone was calling out that they are having to start from scratch at their company to figure out pay ranges, but they don't have the money to potentially buy pay scale data sources. What's their recommendation on how they can begin? Well, the good news is that we have a new freemium offering that is going to be launched shortly, that will be able to give you access to that new explorer experience that Sarah has been talking about today.
Speaker 1:If you want to find out more about that again, click into the poll or reach out to us and we can make sure we can fill you in about that. So that is one way, depending on the number of jobs that you've got to price, obviously, you know, there are freemium options around from some vendors. Sarah, you talked about other free data sources potentially like the BLS data. You know, are other data sources that you can use as well. But I'd also think about this potentially, you know, depending on the number of jobs that you've got to price within your organization from an ROI perspective.
Speaker 1:Because quite often people go, I can't afford to buy pay data. When you need to think about actually what is the impact of not using pay data and the cost to your business potentially of not doing that. You price five jobs wrong and that could actually aggregate up to a value of $2,030,000 US dollars that you're making a mistake on and even more potentially. So, you know, think about framing it from the perspective of the ROI perspective to your organization. And particularly when we're in the slightly cautious macroeconomic environment that we're in at the moment, where we know many organizations are highly cost sensitive, You know, the right pay decision made that is accurate and near term real term data, know, that's really important.
Speaker 1:So think about how you frame that from the kind of ROI perspective to your business. And with that, I think we are at time. So I'm going to ask Amber to come back on stage and close us out. But thank you all very much for your time today. And thank you, Sarah, for sharing your insights.
Speaker 2:Thanks for having me, Ruth.
Speaker 3:Yes, thank you both so much for sharing all of your insights and expertise on this topic. It was a wonderful way to kick off our data series. You will we'll be doing a few more of these events on this topic, covering into different areas over the next couple of months, so stay tuned. Okay. The session today comes in around recording and slides.
Speaker 3:We will send both the recording and slides in one to two business days, as well as some of the follow-up materials that Ruth discussed and some additional ones. So please keep your eye out for those. With that being said, we're out of time. So I will wish you all a wonderful rest of your day, and we hope to see you at a future event soon. Thanks, everyone.
Speaker 1:Thank you.
Creators and Guests
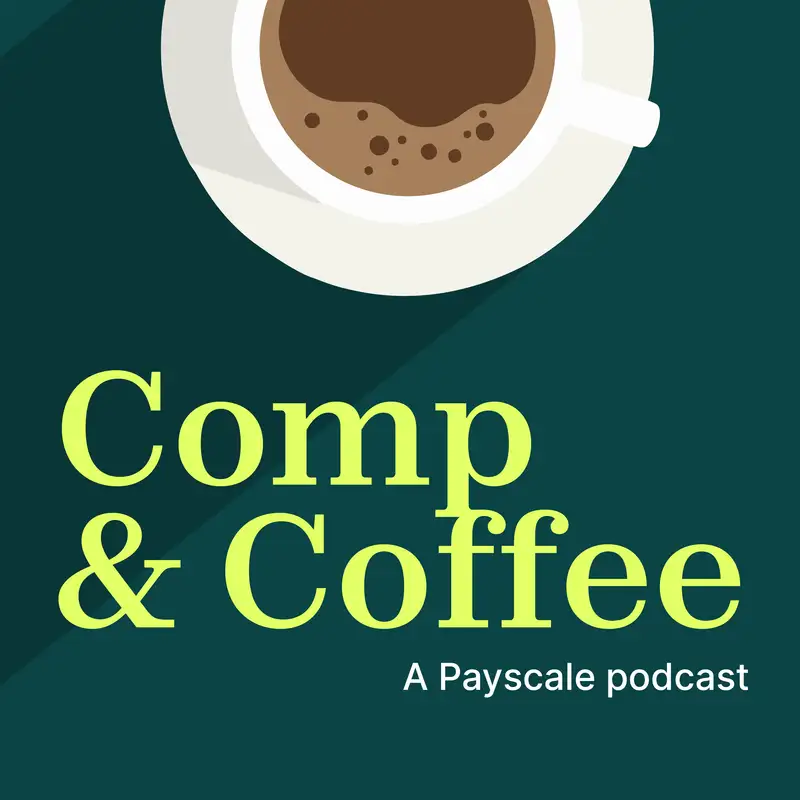